Postdoctoral Research Opportunity in Cloud Computing & Artificial Intelligence (AI) in Agriculture
A complete application consists of:
- An application
- Transcript(s) – For this opportunity, an unofficial transcript or copy of the student academic records printed by the applicant or by academic advisors from internal institution systems may be submitted. All transcripts must be in English or include an official English translation. Click here for detailed information about acceptable transcripts.
- A current resume/CV, including academic history, employment history, relevant experiences, and publication list
- Two educational or professional recommendations
All documents must be in English or include an official English translation.
If you have questions, send an email to USDA-ARS@orau.org. Please include the reference code for this opportunity in your email.
*Applications will be reviewed on a rolling-basis.
Multiple postdoctoral research opportunities are currently available with the U.S. Department of Agriculture (USDA), Agricultural Research Service (ARS) located at one of the regional ARS locations participating in the fellowship program.
This research opportunity is part of the SCINet Fellowship program at ARS. All postdocs will spend time at headquarters for some of their training, but will be based at ARS regional laboratories for more specific training. One of the goals of this research opportunity is to encourage cross-disciplinary, cross-location research; this will be done by placing postdocs in different regional labs based on their skillset and interests in regional locations. The strength of this fellowship program is the collection of postdocs and ARS' collection of regional labs.
The SCINet/Big Data Program at ARS offers research opportunities to motivated postdoctoral participants interested in solving agricultural- and natural resource-related problems at a range of spatial and temporal scales, from the genome to the continent, and sub-daily to evolutionary time scales. One of the goals of the SCINet Initiative is to develop and apply new technologies, including artificial intelligence (AI) and machine learning, to help solve complex agricultural problems that also depend on collaboration across scientific disciplines and geographic locations. In addition, many of these technologies rely on the synthesis, integration, and analysis of large, diverse datasets that benefit from high performance computers (HPC). The objective of these opportunities is to facilitate cross-disciplinary, cross-location research through collaborative research on problems of interest to each participant and amenable to or required by the HPC environment. Training will be provided in specific AI, machine learning, deep learning, and statistical software needed for the HPC.
Predicting the nation’s food supply and ensuring its safety while preserving the natural resources as the environmental drivers continue to change is challenging, and requires integrating and computing vast amounts of high quality data of many different types. Data on U.S. agriculture have been collected since the 1860s. Data streams now include ground-, UAV-, air-, and satellite-based sensors and imagery as well as analyses using genomic data and manually collected data, including animal behavior studies. The advanced computing technologies, such as high performance computing, cloud computing, and AI technologies, needed to fully take advantage of these data are still being developed.
The selected participant will have the opportunity to learn about the challenges in predicting dynamics of agro-ecosystems while learning a range of computational skills needed to conduct these analyses. The participant will also learn AI technologies relevant to these problems, and will contribute to the development of and co-lead ARS-wide workshops resulting in a community of scientific practice with AI. The participant will have the opportunity to collaborate with multiple USDA ARS scientists on data analysis projects, and to write collaborative scientific papers dealing with AI across multiple spatial and temporal scales. These unique research projects will allow candidates the opportunities to apply their programming skills to real-world problems.
Anticipated Appointment Start Date: After October 15, 2019
This program, administered by ORAU through its contract with the U.S. Department of Energy (DOE) to manage the Oak Ridge Institute for Science and Education (ORISE), was established through an interagency agreement between DOE and ARS. The initial appointment is for one year, but may be renewed upon recommendation of ARS and is contingent on the availability of funds. The participant will receive a monthly stipend commensurate with educational level and experience. Proof of health insurance is required for participation in this program. The appointment is full-time at one of the ARS laboratories in the US. Participants do not become employees of USDA, ARS, DOE or the program administrator, and there are no employment-related benefits.
This opportunity is available to U.S. citizens, Lawful Permanent Residents (LPR), and foreign nationals. Non-U.S. citizen applicants should refer to the Guidelines for Non-U.S. Citizens Details page of the program website for information about the valid immigration statuses that are acceptable for program participation.
For more information about the ARS Research Participation Program, please visit the Program Website.
The qualified candidate must have received a doctoral degree in one of the relevant fields before the start date of their appointment.
Preferred skills:
- Experience modeling spatial and/or time series data
- Experience working with large, diverse datasets and data mining approaches
- Proficiency in R and python
- Strong computational skills
- Strong database skills
- Strong oral and written communication skills
- Degree: Doctoral Degree.
- Discipline(s):
- Veteran Status: Veterans Preference, degree received within the last 120 month(s).


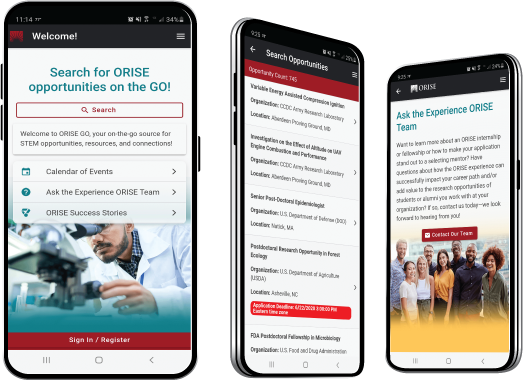
The ORISE GO mobile app helps you stay engaged, connected and informed during your ORISE experience – from application, to offer, through your appointment and even as an ORISE alum!