Create and release your Profile on Zintellect – Postdoctoral applicants must create an account and complete a profile in the on-line application system. Please note: your resume/CV may not exceed 2 pages.
Complete your application – Enter the rest of the information required for the IC Postdoc Program Research Opportunity. The application itself contains detailed instructions for each one of these components: availability, citizenship, transcripts, dissertation abstract, publication and presentation plan, and information about your Research Advisor co-applicant.
Additional information about the IC Postdoctoral Research Fellowship Program is available on the program website located at: https://orise.orau.gov/icpostdoc/index.html.
If you have questions, send an email to ICPostdoc@orau.org. Please include the reference code for this opportunity in your email.
Research Topic Description, including Problem Statement:
With the global diversity of electronic components and printed circuit board (PCB) manufacture and assembly supply chains, there is limited confidence that delivered assembled PCBs have been manufactured and assembled as designed without error. PCB testing is therefore required after manufacturing to verify the PCB functions as required and does not have component(s) missing, wrong component(s) added, component(s) that have failed or will likely fail or components that are counterfeit. In the current climate of a shortage of semiconductor die, the potential of counterfeit components appearing in critical PCBs is increasing. Testing is a trade-off of how extensively to test the product versus time and cost to perform the testing. Depending on the final PCB application the effort to assure the hardware in both secure and insecure environments can be considerable.
Assuring a PCB with components in place is a difficult and manually time-consuming task to perform that does not scale with increasing PCB complexity. Even when original design data or a golden reference PCB are available, generating in-circuit tests and interpreting the results is currently a manually intensive task that relies on engineering best practices and experience to undertake and optimize.
By utilizing parallel world developments in Machine Learning, or other modelling approaches, complex PCBs can be assured against and compared to their specifications. This topic focuses on Machine Learning and modelling methodologies as it is believed this will be key to automating assurance, prior knowledge of PCB development/manufacture is not essential.
Example Approaches:
Possible approach:
- Using high resolution images of PCBs, coupled with component recognition technology and Machine Learning to automate modelling of complex PCBs and their characteristics.
- Model PCBs with components to characterize the resultant measurement values when individual components are interconnected with different values and configurations. Equivalent to measuring the resultant resistance/capacitance/inductance of a PCB net with multiple components attached.
- Use modelling to infer the reverse, decomposing a resultant measurement value into individual component values.
- Develop a model of a PCB optimize the number of measurements required to fully test cover a PCB.
- Develop and build a prediction model to give confidence in assurance level of the PCB
Postdoc Eligibility
- U.S. citizens only
- Ph.D. in a relevant field must be completed before beginning the appointment and within five years of the application deadline
- Proposal must be associated with an accredited U.S. university, college, or U.S. government laboratory
- Eligible candidates may only receive one award from the IC Postdoctoral Research Fellowship Program
Research Advisor Eligibility
- Must be an employee of an accredited U.S. university, college or U.S. government laboratory
- Are not required to be U.S. citizens
Key Words: Machine Learning, Artificial Intelligence, Modelling, Component recognition, Electronics, Hardware Assurance, Printed Circuit Board, Automation, Automated Testing
- Citizenship: U.S. Citizen Only
- Degree: Doctoral Degree.
-
Discipline(s):
- Chemistry and Materials Sciences (12 )
- Communications and Graphics Design (6 )
- Computer, Information, and Data Sciences (17 )
- Earth and Geosciences (21 )
- Engineering (27 )
- Environmental and Marine Sciences (14 )
- Life Health and Medical Sciences (48 )
- Mathematics and Statistics (11 )
- Other Non-Science & Engineering (2 )
- Physics (16 )
- Science & Engineering-related (1 )
- Social and Behavioral Sciences (29 )


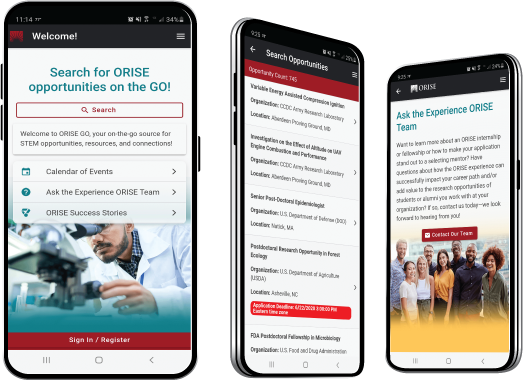
The ORISE GO mobile app helps you stay engaged, connected and informed during your ORISE experience – from application, to offer, through your appointment and even as an ORISE alum!