Needlestacks for Haystacks: Tackling Large-Scale Class-Imbalance
Create and release your Profile on Zintellect – Postdoctoral applicants must create an account and complete a profile in the on-line application system. Please note: your resume/CV may not exceed 2 pages.
Complete your application – Enter the rest of the information required for the IC Postdoc Program Research Opportunity. The application itself contains detailed instructions for each one of these components: availability, citizenship, transcripts, dissertation abstract, publication and presentation plan, and information about your Research Advisor co-applicant.
Additional information about the IC Postdoctoral Research Fellowship Program is available on the program website located at: https://orise.orau.gov/icpostdoc/index.html.
If you have questions, send an email to ICPostdoc@orau.org. Please include the reference code for this opportunity in your email.
Research Topic Description, including Problem Statement:
There is a vast amount of literature around imbalanced learning. Solving imbalanced learning problems is critical in numerous data-intensive networked systems, including surveillance, security, cyber, finance, biomedical, defense, and more. Some recommendations to tackle the class-imbalance problem are collecting more labeled data, changing performance metric, resampling of data, generating synthetic samples, trying various classification algorithms and penalizing the models for mistakes on minority classes. Almost all of these solutions utilize an element of randomization, which leads to different detection outcomes from a single classification algorithm. This topic aims at embedding supervised learning practice in preprocessing to build a deterministic data resampling for the benefit of underlying anomaly detection methods. It is like building a stack of hay-aware needles alongside the existing haystack to hugely increase the chance of picking the lost needle.
Example Approaches:
Under-sampling mainly involves random selection of majority samples to balance them with the minority ones. In contrast, oversampling mostly generates random samples considering the statistics in minority samples to balance them with the majority ones. This topic intends to employ majority statistics plus minority guidelines to train a novel supervised resampling model ahead of conventional classification or anomaly detection phase in the pipeline.
The core idea is that generating augmented minority samples should minimize inter-class variance while maximizing intra-class discrepancy (Fisher Discrimination). Roughly speaking, synthetic samples should mimic both minority and majority patterns to build a high-quality deterministic class-balanced data fed to the classification/detection phase.
Relevance to the Intelligence Community:
Intelligence agencies frequently deal with ‘incomplete’ datasets with few identified targets. Efforts to resolve the imbalanced learning problem may help agencies improve the accuracy of their analytic approaches to identify ‘unknown known’ targets within collected datasets despite the challenges of incomplete data. Real-world intelligence practice deals with few hostile anomalies compared to the large number of legitimate actions. Detection of these anomalies is critical due to the possible damage that they can impose to the national interests and community well-being.
Due to infinitesimal ratio of anomalies to normal behaviors i.e. passengers importing illicit goods vs all other travelers, machine learning techniques usually suffer from class-imbalance syndrome and cannot produce viable detections. This research will address this shortcoming by applying supervised learning to build context-aware class-balanced training data for maximizing detection performance to find needles in haystack.
Key Words: Machine Learning, Imbalanced Learning, Anomaly Detection, Statistics, Synthetic Sampling, Supervised Learning, ML
Postdoc Eligibility
- U.S. citizens only
- Ph.D. in a relevant field must be completed before beginning the appointment and within five years of the application deadline
- Proposal must be associated with an accredited U.S. university, college, or U.S. government laboratory
- Eligible candidates may only receive one award from the IC Postdoctoral Research Fellowship Program
Research Advisor Eligibility
- Must be an employee of an accredited U.S. university, college or U.S. government laboratory
- Are not required to be U.S. citizens
- Citizenship: U.S. Citizen Only
- Degree: Doctoral Degree.
-
Discipline(s):
- Chemistry and Materials Sciences (12 )
- Communications and Graphics Design (2 )
- Computer, Information, and Data Sciences (16 )
- Earth and Geosciences (21 )
- Engineering (27 )
- Environmental and Marine Sciences (14 )
- Life Health and Medical Sciences (45 )
- Mathematics and Statistics (10 )
- Other Non-Science & Engineering (2 )
- Physics (16 )
- Science & Engineering-related (1 )
- Social and Behavioral Sciences (27 )


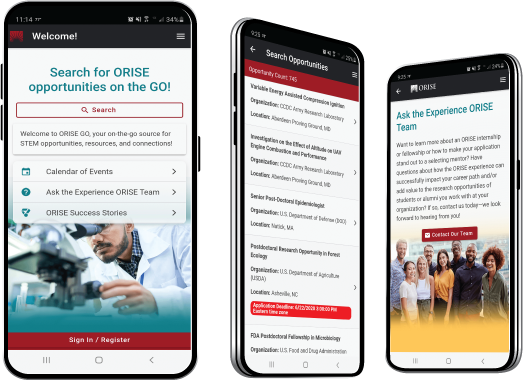
The ORISE GO mobile app helps you stay engaged, connected and informed during your ORISE experience – from application, to offer, through your appointment and even as an ORISE alum!