Low-Shot Training and Testing of Machine Learning Algorithms for Detection of Items of Concern
Create and release your Profile on Zintellect – Postdoctoral applicants must create an account and complete a profile in the on-line application system. Please note: your resume/CV may not exceed 2 pages.
Complete your application – Enter the rest of the information required for the IC Postdoc Program Research Opportunity. The application itself contains detailed instructions for each one of these components: availability, citizenship, transcripts, dissertation abstract, publication and presentation plan, and information about your Research Advisor co-applicant.
Additional information about the IC Postdoctoral Research Fellowship Program is available on the program website located at: https://orise.orau.gov/icpostdoc/index.html.
If you have questions, send an email to ICPostdoc@orau.org. Please include the reference code for this opportunity in your email.
Research Topic Description, including Problem Statement:
X-Ray scanners are deployed for security screening of bags at various security checkpoints in airports, other transport hubs, and at critical national infrastructure.
There is great interest in assessing the effectiveness of machine learning (ML) algorithms deployed on X-Ray scanners to detect concealed items of concern.
To date, it has been considered that a large number and variety of images are required to train an ML algorithm for effective threat detection. However, it may not be possible to provide a large image-set of items of concern to developers. Therefore, it is important to be able to develop effective ML algorithms using a small number of training images—termed low-shot training.
It is also of interest to explore the feasibility of independently testing an algorithm with a small test set of images, termed low-shot testing.
A further option to overcome difficulties with provision of large image-sets of images of concern, is to assess the effectiveness of using synthetic imagery.
Example Approaches:
A small training set of images of items of concern will be provided (open format, not security classified) to the Postdoc Fellow to be used to develop a low-shot trained detection algorithm. A small test set of images will be provided to be used to test the algorithm. A large data set of related images will be provided to train and test an algorithm with a conventional supervised-learning approach. A comparison is to be made of the low-shot learning approach and the supervised-learning approach using the large data set. Similarly, a comparison is to be made of the low-shot testing approach with testing using the large test set.
Based on the provided images of items of concern, the Postdoc Fellow will create synthetic images and develop a study to compare the effectiveness of synthetic images, real images, and a combination of both.
Combine the approaches of low-shot learning and synthetic imagery to assess the most effective way to train an ML algorithm for items of concern in the absence of large data sets.
Relevance to the Intelligence Community:
It is often very difficult or impossible to provide large image sets of items of concern to developers. This may be because these image sets do not exist and would require significant resources of experts and funding to produce; or the images may be security classified. It would, therefore, be advantageous to the Intelligence Community to know if it is possible to effectively train ML algorithms with far fewer images or synthetic images.
Testing the effectiveness of security screening equipment with deployed algorithms is a lengthy, costly process that requires facilities and staff capable of handling hazardous and classified items. A new approach to testing is proposed that, if successful, would make equipment and algorithm testing significantly faster and cheaper. This rapid testing will be essential for the Intelligence Community to keep pace with and exploit the many novel ML algorithms that are anticipated to be developed as this technology matures.
Key Words: Machine Learning, ML, X-Ray Screening, Artificial Intelligence, AI, Low-Shot Learning, Low-Shot Testing, Synthetic Imagery
Postdoc Eligibility
- U.S. citizens only
- Ph.D. in a relevant field must be completed before beginning the appointment and within five years of the application deadline
- Proposal must be associated with an accredited U.S. university, college, or U.S. government laboratory
- Eligible candidates may only receive one award from the IC Postdoctoral Research Fellowship Program
Research Advisor Eligibility
- Must be an employee of an accredited U.S. university, college or U.S. government laboratory
- Are not required to be U.S. citizens
- Citizenship: U.S. Citizen Only
- Degree: Doctoral Degree.
-
Discipline(s):
- Chemistry and Materials Sciences (12 )
- Communications and Graphics Design (2 )
- Computer, Information, and Data Sciences (17 )
- Earth and Geosciences (21 )
- Engineering (27 )
- Environmental and Marine Sciences (14 )
- Life Health and Medical Sciences (45 )
- Mathematics and Statistics (10 )
- Other Non-Science & Engineering (2 )
- Physics (16 )
- Science & Engineering-related (1 )
- Social and Behavioral Sciences (27 )


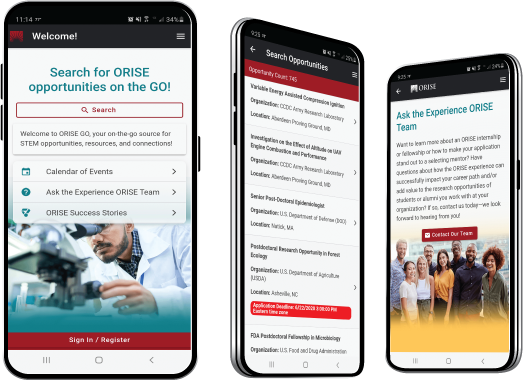
The ORISE GO mobile app helps you stay engaged, connected and informed during your ORISE experience – from application, to offer, through your appointment and even as an ORISE alum!