Artificial Intelligence Explainability
Create and release your Profile on Zintellect – Postdoctoral applicants must create an account and complete a profile in the on-line application system. Please note: your resume/CV may not exceed 2 pages.
Complete your application – Enter the rest of the information required for the IC Postdoc Program Research Opportunity. The application itself contains detailed instructions for each one of these components: availability, citizenship, transcripts, dissertation abstract, publication and presentation plan, and information about your Research Advisor co-applicant.
Additional information about the IC Postdoctoral Research Fellowship Program is available on the program website located at: https://orise.orau.gov/icpostdoc/index.html.
If you have questions, send an email to ICPostdoc@orau.org. Please include the reference code for this opportunity in your email.
Research Topic Description, including Problem Statement:
What is the current state of the art in forensically resolving the provenance of any specific artificial intelligence (AI) result? What is the ability of major AI providers or systems to decompile the data point weighting system in AI algorithms so as to identify how and why an AI/ machine learning (ML) system reached a specific result? What AI development recommendations could or should be made to increase the ability of AI/ML systems to trace how a system reached a given result?
Prospective problem: Social media providers migrating to end-to-end encryption (E2EE) insist in briefings to Congressional staffers that they will be able to identify, block, and report actors engaged in illegal activities, even without access to content, by using AI. A significant issue for law enforcement would become whether a provider’s AI-result report identifying a given subscriber as engaged in criminal activity is of probative value in the probable cause process of seeking and obtaining a search warrant for the subscriber’s residence, where police would hope to gain unfettered access to content through the target’s own computers. The question of legal probative value would likely turn on the government’s ability to prove to a judge that a provider’s AI process produces reliable results in general and produced an accurate result in the specific instance. Stated differently, it would likely turn on the ability of the government to prove how the AI system reached such a conclusion. Whether those AI systems are built to preserve critical information about their own processes could be the determining factor.
At the October 28, 2020 hearing before the U.S. Senate Commerce, Science & Technology Committee, Twitter CEO Jack Dorsey commented on this topic (edited to eliminate repetition):
- “We do agree that we should be publishing more of our practice of content moderation. We have made decisions to moderate content to ensure we are enabling as many voices on our platform as possible. And, I acknowledge, and completely agree with the concerns that it feels like a black box. And, anything we can do to bring transparency to it, including publishing our policies, our practices, answering very simple questions around how our content is moderated, and then doing what we can around the growing trend of algorithms moderating more of this content. This one is a tough one to actually bring transparency to. Explainability in AI is a field of research but it is far out. And I think a better opportunity is giving people more choice around that algorithms they use, including people who turn off the algorithms completely – which is what we are attempting to do.”
Example Approaches:
Some recent computer vision research efforts have endeavored to provide a steppingstone to AI explainability through the use of heat maps. One such example in the facial recognition realm arose from the IARPA JANUS program and is described by Williford et al. in their paper “Explainable Face Recognition” (https://arxiv.org/pdf/2008.00916.pdf). In media forensics, the DARPA MEDIFOR Program (https://www.darpa.mil/program/media-forensics) generated multiple approaches to identifying aspects of images and videos that indicate potential artifacts of manipulation or alteration, sometimes through the use of heat maps, but in other cases without that basic level of explainability. The program manager for MEDIFOR, Matt Turek, now leads the DARPA Explainable Artificial Intelligence (XAI) Program (https://www.darpa.mil/program/explainable-artificial-intelligence), which seeks to develop such capabilities.
Relevance to the Intelligence Community:
The use of AI by the Intelligence Community and law enforcement for decision-making will be limited in scope as long as specific aspects of the process remain obscured in a “black box.” Understanding why specific AI approaches work will give decision-makers confidence that the technology is working in an expected and controlled fashion. Such an understanding will also facilitate better identification of weaknesses in existing processes, which will allow for immediate mitigation of those weaknesses, as well as provide direction for improving the technology. Finally, with better AI explainability, legislators, the judiciary, and the public will gain a higher level of assurance that the technologies being used by the government are fair and equitable.
Key Words: Artificial Intelligence, Machine Learning, Deep Learning, Forensics, AI, ML
Postdoc Eligibility
- U.S. citizens only
- Ph.D. in a relevant field must be completed before beginning the appointment and within five years of the application deadline
- Proposal must be associated with an accredited U.S. university, college, or U.S. government laboratory
- Eligible candidates may only receive one award from the IC Postdoctoral Research Fellowship Program
Research Advisor Eligibility
- Must be an employee of an accredited U.S. university, college or U.S. government laboratory
- Are not required to be U.S. citizens
- Citizenship: U.S. Citizen Only
- Degree: Doctoral Degree.
-
Discipline(s):
- Chemistry and Materials Sciences (12 )
- Communications and Graphics Design (2 )
- Computer, Information, and Data Sciences (16 )
- Earth and Geosciences (21 )
- Engineering (27 )
- Environmental and Marine Sciences (14 )
- Life Health and Medical Sciences (45 )
- Mathematics and Statistics (10 )
- Other Non-Science & Engineering (2 )
- Physics (16 )
- Science & Engineering-related (1 )
- Social and Behavioral Sciences (27 )


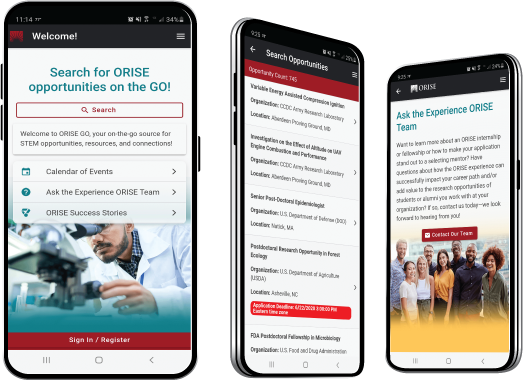
The ORISE GO mobile app helps you stay engaged, connected and informed during your ORISE experience – from application, to offer, through your appointment and even as an ORISE alum!