Formal Verification of Machine Learning Models
Create and release your Profile on Zintellect – Postdoctoral applicants must create an account and complete a profile in the on-line application system. Please note: your resume/CV may not exceed 2 pages.
Complete your application – Enter the rest of the information required for the IC Postdoc Program Research Opportunity. The application itself contains detailed instructions for each one of these components: availability, citizenship, transcripts, dissertation abstract, publication and presentation plan, and information about your Research Advisor co-applicant.
Additional information about the IC Postdoctoral Research Fellowship Program is available on the program website located at: https://orise.orau.gov/icpostdoc/index.html.
If you have questions, send an email to ICPostdoc@orau.org. Please include the reference code for this opportunity in your email.
Research Topic Description, including Problem Statement:
Analysts are increasingly looking to machine learning technologies such as computer vision, natural language processing, and regression analysis to improve the efficiency and effectiveness of a traditionally manual craft. In order to maintain confidence in new approaches, reliable verification methods that can formally demonstrate a program achieves its intended specifications are required. Conventional verification approaches used in system testing and evaluation are insufficient for machine learning algorithms. Because these algorithms are designed to evolve throughout a system’s lifecycle (i.e. the system “learns” from experience), the criteria against which the system should be tested changes constantly, Even algorithms that do not change after deployment, but were produced through machine learning processes are difficult to reliably verify, because they can react unpredictably to inputs that are outside the parameters of their training and test data.
Example Approaches:
- Determining the minimum activity threshold for triggering a change detection algorithm.
- Computing precise geometric bounds on the vulnerabilities of an image classifier to specify the degree and kind of risk from adversarial spoofing.
- Mathematically or quantitatively demonstrating the amount of model drift a text-based learning recommendation engine could be subject to for a legal set of inputs larger than the initial training and test data.
- Using a probabilistic model to ensure the likelihood of classification error remains within a defined limit.
- Calculating the exact error rate for a clustering algorithm using a formal proof instead of empirical tests.
Relevance to the Intelligence Community:
Machine learning programs have the potential to improve analytic practices by automating routine tasks, quickly surfacing and categorizing relevant information, and dynamically monitoring data streams, among other applications. However, because of extremely limited data or intentionally obfuscated information, the Intelligence Community requires the means to estimate how close we think our analysis is to reality and to describe the ways in which we may be wrong. This restraint is further complicated by ethical concerns because analysis informs decisions that may pose risk to lives and national security considerations. Traditional test-and-evaluation and verification-and-validation processes are insufficient for machine learning programs; therefore, new verification approaches and technologies need to be developed to enable the adoption of machine learning models used in analysis.
Key Words: Artificial Intelligence, AI, ML, Machine Learning, Verification, Validation, Testing, Evaluation, Ethics
Postdoc Eligibility
- U.S. citizens only
- Ph.D. in a relevant field must be completed before beginning the appointment and within five years of the application deadline
- Proposal must be associated with an accredited U.S. university, college, or U.S. government laboratory
- Eligible candidates may only receive one award from the IC Postdoctoral Research Fellowship Program
Research Advisor Eligibility
- Must be an employee of an accredited U.S. university, college or U.S. government laboratory
- Are not required to be U.S. citizens
- Citizenship: U.S. Citizen Only
- Degree: Doctoral Degree.
-
Discipline(s):
- Chemistry and Materials Sciences (12 )
- Communications and Graphics Design (2 )
- Computer, Information, and Data Sciences (17 )
- Earth and Geosciences (21 )
- Engineering (27 )
- Environmental and Marine Sciences (14 )
- Life Health and Medical Sciences (45 )
- Mathematics and Statistics (10 )
- Other Non-Science & Engineering (2 )
- Physics (16 )
- Science & Engineering-related (1 )
- Social and Behavioral Sciences (27 )


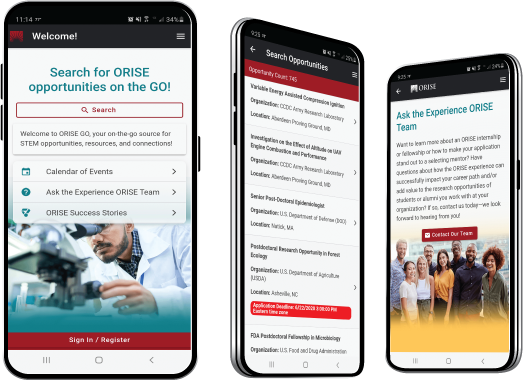
The ORISE GO mobile app helps you stay engaged, connected and informed during your ORISE experience – from application, to offer, through your appointment and even as an ORISE alum!