Explainable and Trustworthy Artificial Intelligence
Create and release your Profile on Zintellect – Postdoctoral applicants must create an account and complete a profile in the on-line application system. Please note: your resume/CV may not exceed 2 pages.
Complete your application – Enter the rest of the information required for the IC Postdoc Program Research Opportunity. The application itself contains detailed instructions for each one of these components: availability, citizenship, transcripts, dissertation abstract, publication and presentation plan, and information about your Research Advisor co-applicant.
Additional information about the IC Postdoctoral Research Fellowship Program is available on the program website located at: https://orise.orau.gov/icpostdoc/index.html.
If you have questions, send an email to ICPostdoc@orau.org. Please include the reference code for this opportunity in your email.
Research Topic Description, including Problem Statement:
The adaptation of Artificial intelligence (AI) capabilities is necessary for analytical insights into big data to reach its full potential. A key barrier to adoption appears to be user trust in ‘black-box’ algorithms, especially under uncertainty and when dealing with high-stakes consequences. A thorough understanding of user trust, interpretability of AI techniques and design methodologies for assistant uptake and trust in these systems is imperative if these technological advantages are to be adopted in order to achieve required analytical outcomes.
The issue of explainable AI remains a major obstacle to the broader application of AI-powered products and services due to issues of transparency and accountability. In addition to public debate around the need for transparency and accountability to be built into AI applications, this issue remains an obstacle for governments developing regulatory frameworks and legislative changes to govern the use of AI technologies. Many engineers and data scientists have questioned whether meaningful explainability, to the degree required, is technically possible, particularly as approaches such as neural networks and deep learning are becoming increasingly ubiquitous and complex.
Example Approaches:
Research proposals could approach this from a variety of disciplines, or as a cross-disciplinary effort. The problem touches on aspects of data science, engineering, psychology, human-centered computing, systems and design thinking, software development and UX and UI design, with links into social sciences.
Proposals could consider:
- Explainable AI and approaches for increasing the transparency and reasoning behind more complex deep learning methods.
- Understanding the antecedents to trust and propensities user have to trust new technological capabilities.
- Understanding barriers to user trust and how to regain trust once lost.
- How the measured consideration of design of systems could influence trust and how system features may be manipulated to mitigate loss of trust.
- How human-machine interfaces can be better designed to enable a symbiotic working relationship between the human and computer.
Relevance to the Intelligence Community:
As data volumes and complexities increase and become more difficult to analyze manually, AI capabilities will need to be adopted to produce critical results in any meaningful timeframe. It is imperative that the IC understand user trust in new technological capabilities and designs AI systems accordingly in order to facilitate trust amongst end-users, and ensure systems can recover when perceived trust is lost.
Deep learning and neural networks are built into the core of many applications and capabilities of high interest and worth to intelligence services. The ‘black box’ problem remains perhaps the best known issue commonly discussed in public discourse about AI and machine learning. The issues raise concerns around the ethics and accountability of AI applications, particularly where applied to counter security threats and challenges. Improving the explainability of neural networks would assist developers to refine and improve neural networks applications. Additionally, improvements in the accountability of AI solutions would support public trust in AI, particularly within government AI capabilities and solutions.
Key Words: Artificial Intelligence, Trust In Technology, Automation, Uncertainty, Explainable AI, Trusted Analytics
Postdoc Eligibility
- U.S. citizens only
- Ph.D. in a relevant field must be completed before beginning the appointment and within five years of the application deadline
- Proposal must be associated with an accredited U.S. university, college, or U.S. government laboratory
- Eligible candidates may only receive one award from the IC Postdoctoral Research Fellowship Program
Research Advisor Eligibility
- Must be an employee of an accredited U.S. university, college or U.S. government laboratory
- Are not required to be U.S. citizens
- Citizenship: U.S. Citizen Only
- Degree: Doctoral Degree.
-
Discipline(s):
- Chemistry and Materials Sciences (12 )
- Communications and Graphics Design (2 )
- Computer, Information, and Data Sciences (16 )
- Earth and Geosciences (21 )
- Engineering (27 )
- Environmental and Marine Sciences (14 )
- Life Health and Medical Sciences (45 )
- Mathematics and Statistics (10 )
- Other Non-Science & Engineering (2 )
- Physics (16 )
- Science & Engineering-related (1 )
- Social and Behavioral Sciences (27 )


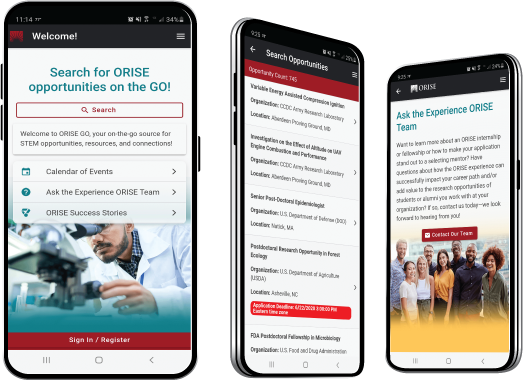
The ORISE GO mobile app helps you stay engaged, connected and informed during your ORISE experience – from application, to offer, through your appointment and even as an ORISE alum!