Using Eigenbehaviors To Project Psychological Profiles Of Groups And Individuals
Create and release your Profile on Zintellect – Postdoctoral applicants must create an account and complete a profile in the on-line application system. Please note: your resume/CV may not exceed 2 pages.
Complete your application – Enter the rest of the information required for the IC Postdoc Program Research Opportunity. The application itself contains detailed instructions for each one of these components: availability, citizenship, transcripts, dissertation abstract, publication and presentation plan, and information about your Research Advisor co-applicant.
Additional information about the IC Postdoctoral Research Fellowship Program is available on the program website located at: https://orise.orau.gov/icpostdoc/index.html.
If you have questions, send an email to ICPostdoc@orau.org. Please include the reference code for this opportunity in your email.
Research Topic Description, including Problem Statement:
Personality traits and cognitive intelligence can help identify and explain human behaviors. However, the reconstruction of a psychological profile from activity to behavior, from behavior to personality and/or cognitive traits has never been attempted. Current research using eigenbehaviors to identify human activities and movements can only be seen at the group level1, i.e. affiliation, relationships, similarity between individuals, but not at the individual level.
Location data, correspondence, social media platform data, etc. provide a unique look at human behavior in the form of eigenbehaviors. These eigenbehaviors represent both societal/cultural influences (i.e. external factors) as well as individual influences driven by an individual’s unique psychological makeup2. This topic supports research into improving methods for generating the eigenvectors representing eigenbehaviors using multi-modal data (location information, audio cues, textual correspondence, etc.) and using these resultant vectors to extract information corresponding to a psychological assessment of the individual.
Example Approaches:
Using the available modalities do one of the following; generate summary statistics about the modalities for shorter intervals and keep the overall vector interval to a day (similar to Pentland et al.3), or generate summary statistics for the entire chosen time interval (say a day) and use the at the vector. Post this processing the remainder of the approach used in Pentland is the same.
The second possible approach is more nuanced and potentially complex. It involves developing a tokenization scheme based on the available modalities and generating tokenized patterns or vectors for say a day of the subject’s activity. At this point Pentland et al.’s methodology needs to be modified since we cannot easily subtract out a baseline behavioral sequence (i.e. the average behavioral vector). It would be necessary to investigate whether this is still necessary before proceeding with the eigenvector decomposition.
Regardless of which approach is chosen for producing the eigenvectors/eigenbehaviors improved methods are needed for analyzing these outcomes. Pentland et al. and others have clustered the behaviors into groups and therefore constrained themselves to group level classification. Since we are concerned with the subject’s psychological makeup, clustering is not an apropos approach, instead we need to consider classification approaches. Proposers are encouraged to leverage publicly available datasets for their investigation.
Relevance to the Intelligence Community:
The primary focus of existing research in inferring individual behaviors and establishing/predicting behavioral patterns has focused on single modality data sources and methodologies. This has, inadvertently, created a gap between the analysis of group (individuals who generally act the same way) and individual behavior at scale. While the Intelligence Community is interested in country scale behavioral dynamics, we also seek to better understand the subject level behaviors that inform and drive those higher level dynamics. This research seeks to resolve that gap in two ways. First, explore methodologies for benefiting from the additional information other modalities provide. Second, explore generalization of these methodologies to enable rapid incorporation of new data sources in the future. This work will take a step toward resolving/informing future research and development.
References:
- Eagle, N, & Pentland, A. (2009). Eigenbehaviors: Identifying Structure in Routine. Behavioral Ecology and Sociobiology, 63, 1057-1066.
- Schiavone, G., Lamichhane, B., & Van Hoof, C (2017). The Double Layer Methodology and the Validation of Eigenbehavior Techniques Applied to Lifestyle Modeling. BioMed Research International, 2017(1), 1-15.
- Pentland, A. (2007). On the Collective Nature of Human Intelligence. International Society for Adaptive Behavior, 15(2), 189-198.
Key Words: Eigenbehaviors, Eigendecomposition, Episodic Features, Psychological Traits, Behavioral Modeling
Postdoc Eligibility
- U.S. citizens only
- Ph.D. in a relevant field must be completed before beginning the appointment and within five years of the application deadline
- Proposal must be associated with an accredited U.S. university, college, or U.S. government laboratory
- Eligible candidates may only receive one award from the IC Postdoctoral Research Fellowship Program
Research Advisor Eligibility
- Must be an employee of an accredited U.S. university, college or U.S. government laboratory
- Are not required to be U.S. citizens
- Citizenship: U.S. Citizen Only
- Degree: Doctoral Degree.
-
Discipline(s):
- Chemistry and Materials Sciences (12 )
- Communications and Graphics Design (2 )
- Computer, Information, and Data Sciences (16 )
- Earth and Geosciences (21 )
- Engineering (27 )
- Environmental and Marine Sciences (14 )
- Life Health and Medical Sciences (45 )
- Mathematics and Statistics (10 )
- Other Non-Science & Engineering (2 )
- Physics (16 )
- Science & Engineering-related (1 )
- Social and Behavioral Sciences (27 )


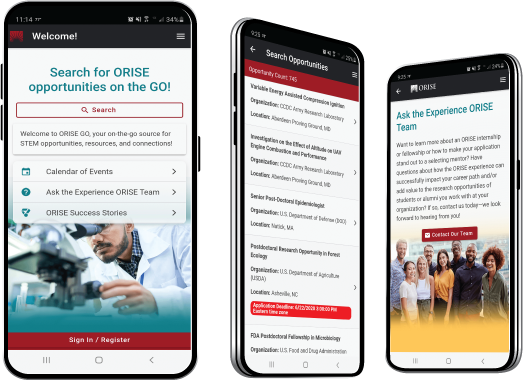
The ORISE GO mobile app helps you stay engaged, connected and informed during your ORISE experience – from application, to offer, through your appointment and even as an ORISE alum!