Use of artificial intelligence to validate data and to automate correction of poor-quality data
Create and release your Profile on Zintellect – Postdoctoral applicants must create an account and complete a profile in the on-line application system. Please note: your resume/CV may not exceed 2 pages.
Complete your application – Enter the rest of the information required for the IC Postdoc Program Research Opportunity. The application itself contains detailed instructions for each one of these components: availability, citizenship, transcripts, dissertation abstract, publication and presentation plan, and information about your Research Advisor co-applicant.
Additional information about the IC Postdoctoral Research Fellowship Program is available on the program website located at: https://orise.orau.gov/icpostdoc/index.html.
If you have questions, send an email to ICPostdoc@orau.org. Please include the reference code for this opportunity in your email.
Research Topic Description, including Problem Statement:
- Presently there are a number of law enforcement systems that are used for logging and to make enquiries pertaining to a potential suspect. The data held by systems may contain inaccuracies, duplication, redundant information and become outdated. This can be the result of misspelt and non-validated entries, which is then stored and retrieved from systems. Due to erroneous data, users will attempt to insert new entries to rectify the inaccuracies, thus contributing to the disparate amount of information held on the one suspect. From an analytics’ perspective erroneous data creates difficulties when comparing entries from multiple systems, as there may be multiple unique identifiers generated for the one suspect due to incorrect data. To deliver accurate and effective analytics, error correction and de-deduplication are generally carried out manually by an analyst who will need to validate the entries. This is often time consuming, laborious and is an inefficient use of the analyst’s time due to the volume of entries and resources needed to comb through the data.
- The advances of Artificial Intelligence (AI) and automation have the potential to greatly enhance our information sharing, processing and analytics capabilities. We believe that research and exploration into these technologies is essential for improving confidence in our own data, which could be used in future to explore options such as analytics for predictive policing, where the outcomes are dependent on the quality of data.
Example Approaches:
- Stage 1: Improve textual, Global Positioning System (GPS) and multimedia data submission, validation and verification of existing law enforcement systems.
- Outputs include a comparison when a tasking is assigned on how will we know if:
- The system has implemented the error corrections in comparison to the human analyst
- There is a difference in productivity, that is has the quality of tasking improved due to the error corrections and suggestions made by the system?
- Outputs include a comparison when a tasking is assigned on how will we know if:
- Stage 2: Data washing/cleansing/enrichment: Exploring and suggestion of an “intelligent” way to automate the process of erroneous data which an analyst would be expected to clean up and ensure data is fit for purpose.
- Examine the use of automated and supervised/semi supervised learning approaches to learn corrections that should be applied to the data.
- Confidence and error rate of the algorithm in comparison to a human analyst, for example trials to see whether a machine would be more accurate in their corrections and suggestions.
- Data provenance such as the origins of the data. How has the algorithm/automated technique/method arrived at that decision?
- Stage 3: Repetition of the process but with multiple data feeds from a variety of law enforcement systems.
- Outputs would be the same as above.
- Inference of the data. Outputs could include deriving one “joined up” unique identifier purporting to that individual and seeking out any redundancies to be corrected and potentially merged.
- Stage 4: Early proof of concept demonstrator to determine the feasibility of outputs and any demonstrable benefits of implementing intelligent error correction. This will also be used to feedback and inform stage 5 work (below) as appropriate.
- Stage 5: Repetition of the process with other shared law enforcement databases.
- Outputs would be the same as above.
- Stage 6: Introduction of modules to include open source data that is available in the public domain, for example: weather, company, land registration and census.
- It is envisaged that this topic could be approached by:
- A review of current academic/research literature;
- Testing of the technology capability;
- Proof-of-concept demonstrator.
Key Words:
Data; Artificial Intelligence; Automation; Validation; Machine Learning; Analytics; Error Correction; Knowledge; Semi-Supervised; Supervised; Big Data; Search; Prediction; Data Cleansing; Data Enrichment; Deep Learning; Data Formatting; Data Processing; Natural Language Processing; Neural Network.
Postdoc Eligibility
- U.S. citizens only
- Ph.D. in a relevant field must be completed before beginning the appointment and within five years of the application deadline
- Proposal must be associated with an accredited U.S. university, college, or U.S. government laboratory
- Eligible candidates may only receive one award from the IC Postdoctoral Research Fellowship Program.
Research Advisor Eligibility
- Must be an employee of an accredited U.S. university, college or U.S. government laboratory
- Are not required to be U.S. citizens
- Citizenship: U.S. Citizen Only
- Degree: Doctoral Degree.
-
Discipline(s):
- Chemistry and Materials Sciences (12 )
- Communications and Graphics Design (6 )
- Computer, Information, and Data Sciences (16 )
- Earth and Geosciences (21 )
- Engineering (27 )
- Environmental and Marine Sciences (14 )
- Life Health and Medical Sciences (45 )
- Mathematics and Statistics (10 )
- Other Non-Science & Engineering (5 )
- Physics (16 )
- Science & Engineering-related (1 )
- Social and Behavioral Sciences (28 )


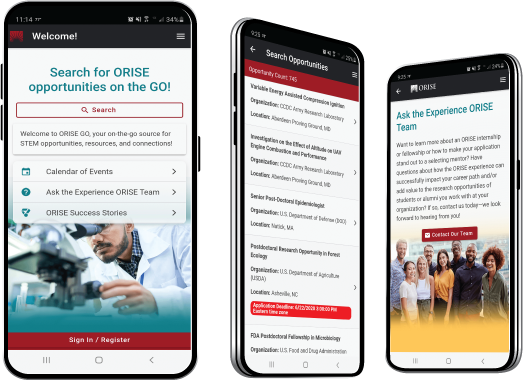
The ORISE GO mobile app helps you stay engaged, connected and informed during your ORISE experience – from application, to offer, through your appointment and even as an ORISE alum!