Machine Learning for Identifying/Locating Natural Targets Using Multi-temporal Dual-Pol SAR Data
Create and release your Profile on Zintellect – Postdoctoral applicants must create an account and complete a profile in the on-line application system. Please note: your resume/CV may not exceed 2 pages.
Complete your application – Enter the rest of the information required for the IC Postdoc Program Research Opportunity. The application itself contains detailed instructions for each one of these components: availability, citizenship, transcripts, dissertation abstract, publication and presentation plan, and information about your Research Advisor co-applicant.
Additional information about the IC Postdoctoral Research Fellowship Program is available on the program website located at: https://orise.orau.gov/icpostdoc/index.html.
If you have questions, send an email to ICPostdoc@orau.org. Please include the reference code for this opportunity in your email.
Research Topic Description, including Problem Statement:
- Despite the nature of coherent speckle noise and geometric distortions of synthetic aperture radar (SAR) data, automatic target recognition (ATR) from SAR images has been extensively studied due to its practical use in both military and civil applications. There remain some unresolved problems in these applications. The SAR ATR application is primarily used to recognize artificially-constructed objects rather than natural targets such as crops. The objective of this research use deep learning algorithms to identify and locate crops in space borne SAR images (Sentinel-1) with resolution less than airborne sensors and with minimal or no ground truth. The SAR data only collects in VV and VH polarizations.
- The SAR ATR procedure is typically composed of three steps: preprocessing, feature extraction, and classification. In the past, handcrafted features were first extracted from images using feature descriptors. The accuracy of the classification is profoundly dependent on the design of the feature extraction. In recent years, deep convolutional neural networks (DCNN) have achieved great success in image classification and recognition, and in many other applications due to their capability to learn features from data without task-specific feature extractors. Many DCNN SAR ATR studies validate their algorithms using the Moving and Stationary Target Acquisition and Recognition (MSTAR) dataset jointly sponsored by the Defense Advanced Research Projects Agency (DARPA) and Air Force Research Laboratory (AFRL).The MSTAR dataset contains image chips of tanks, infantry fighting vehicles, armored personnel carriers and multiple simple geometric shaped static targets. However, for natural targets, such as crops, the researchers must rely on field experiments to collect ground truth for training and validation. A few researchers reported in the literature that their DCNN works for crop classification using full polarization JPL AIRSAR data over Flevoland, a flat farmland in the Netherlands. The Flevoland ground truth was collected for the JPL-SAR and ERS-1 Campaign that took place in 1991.Another study using deep learning (recurrent neural network) and multi-temporal space borne SAR (Sentinel-1) data for agricultural land cover map was carried out at Camargue, France with available field survey data of over 921 reference plots. Under most circumstances, researchers will not have this type of information.
- Under the assumption of little or no ground truth, one can consider that time series datasets provide spatio-temporal presentations for DCNN for crop or vegetation classification. Benefiting from high-quality repeat orbit dual-polarization (dual-pol) SAR images captured by Earth observation satellites, such as Sentinel 1, this time series of dual-pol SAR data can be transformed into discriminative feature vectors for crop classification. The spatio-temporal approach is becoming a fundamental technology for crop identification and yield assessment.
Example Approaches:
- An unclassified example that uses EO data instead of SAR can be found in Remote Sens. 2018, 10, 75; titled “3D Convolutional Neural Networks for Crop Classification with Multi-Temporal Remote Sensing Images.” written by Ji, S., et al.That study suggested a three-dimensional (3D) convolutional neural networks (CNN) based method that automatically classified crops from spatio-temporal remote sensing images. First, a 3D kernel was designed according to the structure of multi-spectral multi-temporal remote sensing data. Secondly, the 3D CNN framework with fine-tuned parameters was designed for training 3D crop samples and learning spatio-temporal discriminative representations, and preserving the full crop growth cycles. In addition, they introduced an active learning strategy to the CNN model to improve labelling accuracy to a required threshold with the most efficiency. Finally, experiments were carried out to test the advantage of the 3D CNN, compared to the two-dimensional (2D) CNN and other conventional methods. Their experiments showed that the 3D CNN was especially suitable in characterizing the dynamics of crop growth and outperformed the other mainstream methods.
Relevance to the Intelligence Community
- Analysis on illicit drug production and transnational drug trafficking that threaten US national security are important operations for counter narcotics. Improving the capability of estimating cultivation acreage of a wide range of illicit crops in nations that are known for growing specific illicit crops will be increasingly necessary research in the intelligence community.
Key Words: SAR, Machine Learning, Dual-Polarization, Neural Networks
Postdoc Eligibility
- U.S. citizens only
- Ph.D. in a relevant field must be completed before beginning the appointment and within five years of the application deadline
- Proposal must be associated with an accredited U.S. university, college, or U.S. government laboratory
- Eligible candidates may only receive one award from the IC Postdoctoral Research Fellowship Program.
Research Advisor Eligibility
- Must be an employee of an accredited U.S. university, college or U.S. government laboratory
- Are not required to be U.S. citizens
- Citizenship: U.S. Citizen Only
- Degree: Doctoral Degree.
-
Discipline(s):
- Chemistry and Materials Sciences (12 )
- Communications and Graphics Design (6 )
- Computer, Information, and Data Sciences (16 )
- Earth and Geosciences (21 )
- Engineering (27 )
- Environmental and Marine Sciences (14 )
- Life Health and Medical Sciences (45 )
- Mathematics and Statistics (10 )
- Other Non-Science & Engineering (5 )
- Physics (16 )
- Science & Engineering-related (1 )
- Social and Behavioral Sciences (28 )


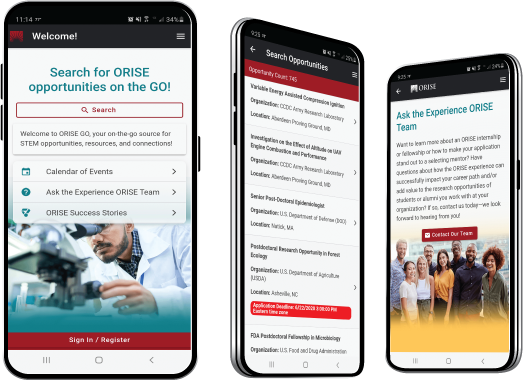
The ORISE GO mobile app helps you stay engaged, connected and informed during your ORISE experience – from application, to offer, through your appointment and even as an ORISE alum!