Adversarial Biological Learning
Create and release your Profile on Zintellect – Postdoctoral applicants must create an account and complete a profile in the on-line application system. Please note: your resume/CV may not exceed 2 pages.
Complete your application – Enter the rest of the information required for the IC Postdoc Program Research Opportunity. The application itself contains detailed instructions for each one of these components: availability, citizenship, transcripts, dissertation abstract, publication and presentation plan, and information about your Research Advisor co-applicant.
Additional information about the IC Postdoctoral Research Fellowship Program is available on the program website located at: https://orise.orau.gov/icpostdoc/index.html.
If you have questions, send an email to ICPostdoc@orau.org. Please include the reference code for this opportunity in your email.
Research Topic Description, including Problem Statement:
- In adversarial machine learning, an artificial neural network (ANN) is trained to classify images or other data at a high level of accuracy, but an attacker manipulates the image very subtly, which causes the ANN to misclassify the image with a high degree of confidence. There are biological analogues to adversarial machine learning. A biological neural network (BNN) can be fooled through camouflage, optical illusions or confirmation bias and make mistakes. Images have been manipulated to fool both ANNs and BNNs, (in this case, namely humans). However, whether the manipulations fool humans only marginally and briefly or the manipulations do damage to the semantics behind the image; the manipulated image “truly” conveys something else, even after considered human judgment.
- Can more robust attacks against BNNs be created, which do not destroy the “true” semantics of the inputs? Why or why not? What is the right way to quantify damage to an inputs’ semantics when the ground truth of the inputs is derived from the assessments of humans (which are BNNs)? Is the robustness or weakness of BNNs to adversarial attacks specific to image recognition, or does it extend to all modalities? Are the principles of adversarial attacks on BNNs different from those on ANNs? How can BNNs be made more robust to defend against such attacks?
Example Approaches:
While we are open to all approaches, we expect that first steps might include:
- constructing BNNs,
- training them to classify images or other inputs,
- attempting to create methods to manipulate inputs so that the BNNs robustly misclassify them
- inspecting the BNNs to see what internal factors affect robustness
- developing means to quantify the size of the semantic damage done to the inputs, which may require describing the manipulations in a basis that maps to the BNNs’ information processing patterns
Notably, there are multiple ways to construct these BNNs, including:
- In vitro with neuron cell cultures or slices
- In vivo with model species (perhaps leveraging recordings or stimulation of BNNs with non-invasive or invasive methods)
- In silico methods with detailed physiological models of BNNs, to be contrasted with the simpler ANNs currently used in machine learning.
Relevance to the Intelligence Community:
In order for the IC to use automated classifiers to sift through mountains of data, those classifiers must be robust to the array of startlingly simple attacks that would allow an adversary to fool the classifier. Fortunately, a growing segment of the academic machine learning community is studying adversarial machine learning attacks and how to mitigate them. However, there is as yet no known research examining if that new line of research is relevant to biology. If these techniques can be leveraged to fool human analysts or operators in novel ways, that would put intelligence collection efforts in jeopardy and create counterintelligence opportunities. Perhaps more probably, examining how BNNs are robust to adversarial attacks may reveal how to make machine classifiers more robust, making automated collection efforts resistant to adversarial attacks.
Key Words: Adversarial machine learning, neuroscience, learning, biology, attack
Postdoc Eligibility
- U.S. citizens only
- Ph.D. in a relevant field must be completed before beginning the appointment and within five years of the application deadline
- Proposal must be associated with an accredited U.S. university, college, or U.S. government laboratory
- Eligible candidates may only receive one award from the IC Postdoctoral Research Fellowship Program.
Research Advisor Eligibility
- Must be an employee of an accredited U.S. university, college or U.S. government laboratory
- Are not required to be U.S. citizens
- Citizenship: U.S. Citizen Only
- Degree: Doctoral Degree.
-
Discipline(s):
- Chemistry and Materials Sciences (12 )
- Communications and Graphics Design (6 )
- Computer, Information, and Data Sciences (16 )
- Earth and Geosciences (21 )
- Engineering (27 )
- Environmental and Marine Sciences (14 )
- Life Health and Medical Sciences (45 )
- Mathematics and Statistics (10 )
- Other Non-Science & Engineering (5 )
- Physics (16 )
- Science & Engineering-related (1 )
- Social and Behavioral Sciences (28 )


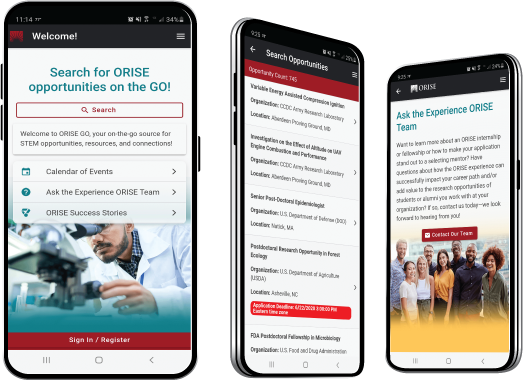
The ORISE GO mobile app helps you stay engaged, connected and informed during your ORISE experience – from application, to offer, through your appointment and even as an ORISE alum!